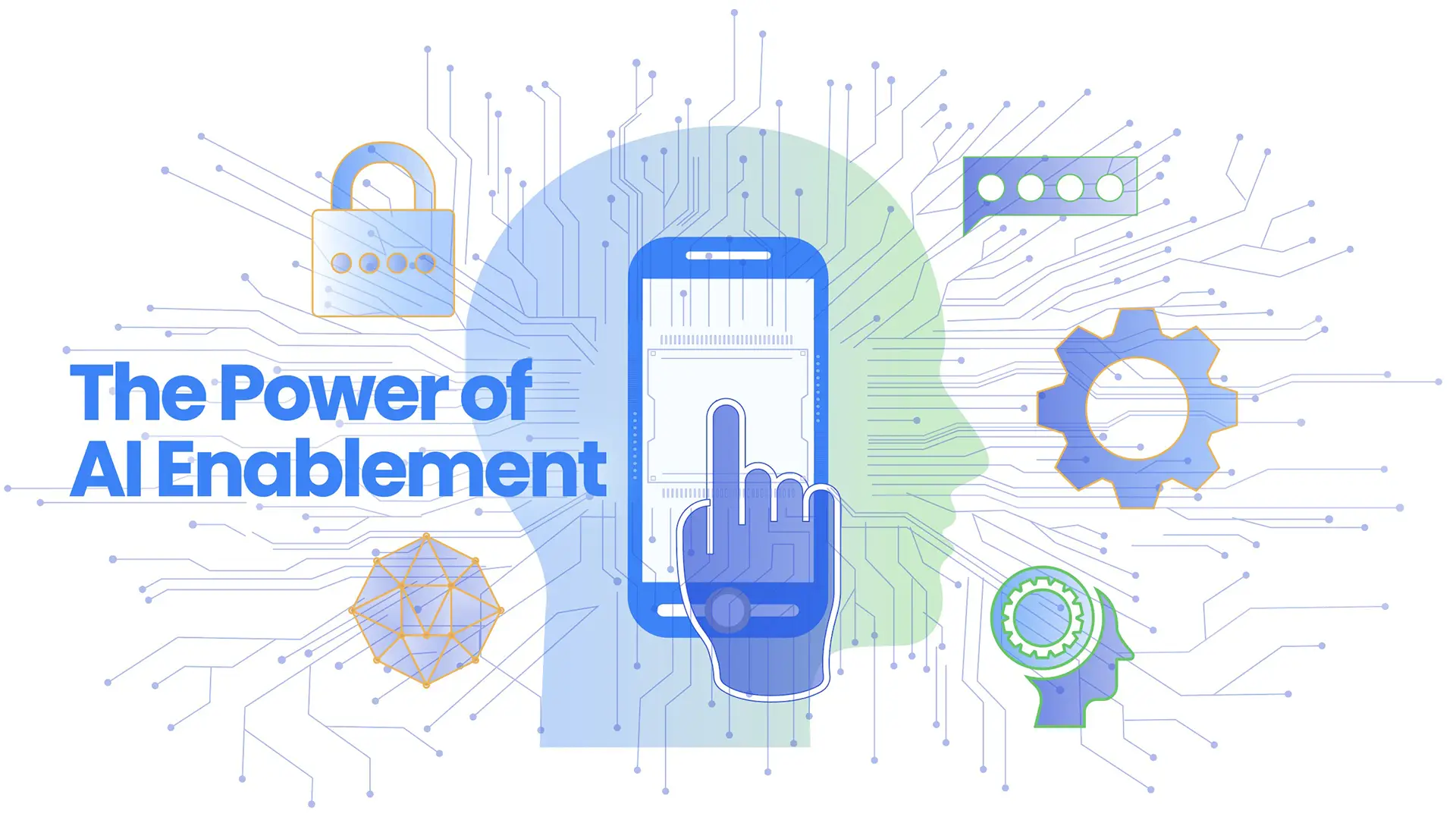
A More Pragmatic and Profitable Approach
2022 marked the year when the public began witnessing significant advancements in AI research, transitioning from the lab to real-world applications. ChatGPT, in just two months, educated over 100 million people globally about transformers. What was once a budding area of research has now become the next major platform shift in technology. The question now is: How best can companies leverage AI to transform their businesses and operations?
AI companies can be broadly divided into two categories: AI-First and AI-Enabled.
- AI-First companies have artificial intelligence at the core of their business model, requiring deep AI research, significant domain expertise, and substantial capital. Investors in these companies often adopt a long-term view, as viable business models tend to be unconventional and require extended development timelines.
- AI-Enabled companies emphasize customer issues and leverage AI to enhance or optimize processes, operations and products to transform businesses.
At ECSite, we’ve chosen to adopt an AI-Enabled approach, which we believe can have a far-reaching and more immediate impact than AI-First strategies. Here are the key reasons why we’ve committed to this path and how it’s shaping our success:
1. AI-Enabled creates a stronger moat and is lower cost
AI-First companies often invest heavily in research and development, pouring money into building and training complex models. This process demands vast data sets, extensive training, and AI researchers, all contributing to high costs. However, with rapid advancements in model building, it’s becoming easier for other vendors to achieve similar, if not better, results using the same data sets.
Differentiation between models is diminishing. For instance, hundreds of startups now offer conversational interfaces for multimodal data, many based on generative AI, either as core features or add-ons. This makes it increasingly difficult for SaaS companies to monetize their R&D costs. In contrast, data becomes the key moat for AI-Enabled companies, allowing them to leverage existing models and focus their AI offerings on tailored solutions.
2. Rapid Commoditization of AI
One of the driving forces behind the commoditization of AI, particularly in generative AI, is the lack of significant differentiation among foundational models. Without standout features, pricing faces downward pressure. Even as demand grows, competition pushes prices lower. Open-source models, such as Llama 3, complicate vendor pricing strategies further.
While companies like OpenAI and Anthropic have introduced cost-saving measures like prompt caching and batching, they are still facing massive losses—$5 billion annually for OpenAI and $2.7 billion for Anthropic. The lack of differentiation, combined with high operational costs, means these companies may need to adopt new pricing strategies. As the industry consolidates, specialized language models are also likely to face similar challenges, making it harder to deliver truly unique solutions.
3. Playing to Our Strengths
At ECSite, we made a deliberate decision to focus on our strengths rather than chasing the AI-First trend. This strategy has proven highly beneficial. With over a petabyte of customer-labeled data across our app ecosystem, we’ve built a robust AI-powered infrastructure. Through reinforcement learning and fine-tuning large language models (LLMs) to meet the specific needs of our customers, we’ve managed to seamlessly integrate AI into our core products—at a fraction of the cost.
This approach has strengthened our solutions and paved the way for monetizing AI, leading to increased revenue per customer. Our extensive data resources have enhanced our services, streamlined workflows, and generated a data flywheel effect that continues to drive growth.
The Power of 80% Solutions
Through experimentation, we’ve discovered that solving 80% of the use cases with at least 80% accuracy in AI models can be extremely beneficial in the immediate term. This approach enables us to deliver immediate value to our customers, while continuously working with them to fine-tune accuracy as needed. Here’s what this strategy has helped us achieve:
- Cost Reduction: By targeting a more attainable accuracy level, we’ve significantly reduced the capital required for AI development and deployment. This allows us to allocate resources more efficiently.
- Faster Deployment: With shorter development timelines, we’re able to implement AI solutions more quickly, enabling us to realize benefits sooner.
A Sustainable Path to Growth
Our AI-Enabled strategy aligns perfectly with our business needs, enhancing our core product offerings and providing a clear path to monetization. By focusing on value-driven, tailored solutions, we’ve differentiated ourselves from AI-First competitors. This approach helps us avoid the risks of pricing pressure and commoditization, ensuring sustainable growth in an increasingly competitive market.
At ECSite, we are continually expanding our four key AI-Enabled use cases: the AI QC module, Anomaly Detection, Edge AI, and AskVina™ (*the world’s first conversational interface for digital infrastructure deployment). To learn more, feel free to reach out to our sales or customer success teams.
In a world where many companies are rushing to go AI-First, we’ve chosen a different route—one that focuses on what works for us and, most importantly, what works for our customers and investors.